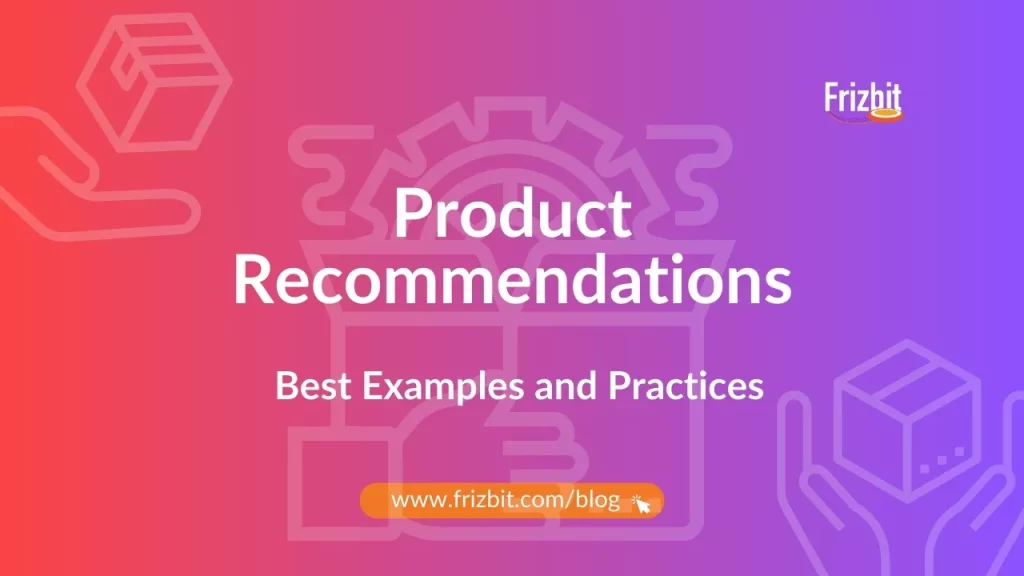
Implementing product recommendations in the eCommerce industry is essential for delivering personalised shopping experiences.
These recommendations not only enhance user engagement but also drive sales, foster customer loyalty, and increase revenue by up to 10%.
This guide delves into the most effective examples of product recommendations and the best practices for implementing them effectively, providing a solid foundation before starting to work with a product recommendation engine.
Product Recommendations Examples – Brands Doing It Right!
Frequently Bought Together
This type of product recommendations suggest items that are often purchased together by other customers, leveraging data from previous transactions to identify patterns in purchasing behaviour.
For example, if many customers who buy a dumbbell also purchase a workout mat and a set of resistance bands, Amazon will recommend these additional items to someone viewing the dumbbell product page.
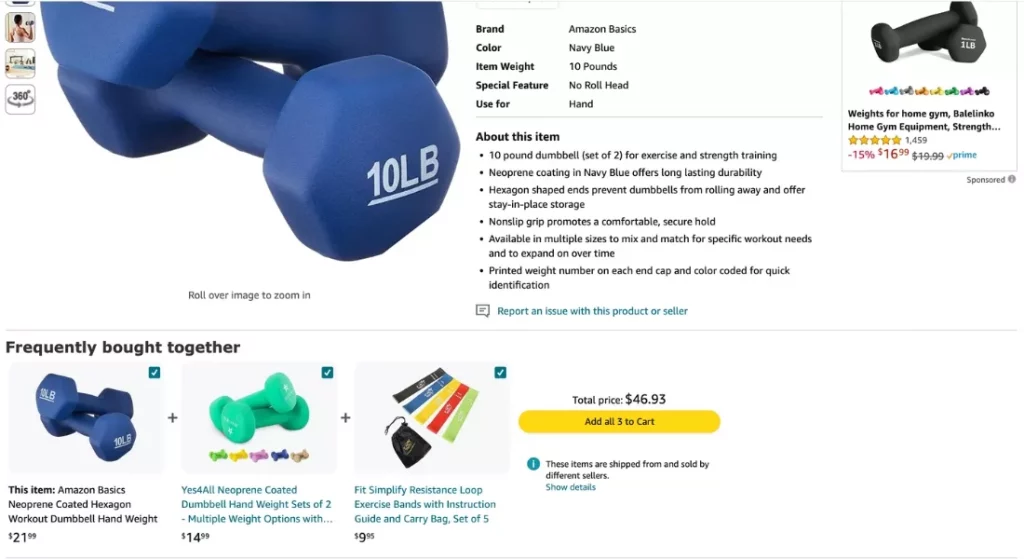
Pros and Cons:
Pros: Increases the average order value by encouraging customers to buy complementary products. Enhances the overall shopping experience by providing useful suggestions.
Cons: Requires accurate data to ensure the recommendations are relevant, which can be resource-intensive to maintain.
Similar Items
Similar Items product recommendations feature suggests that items that are alike in nature to the one a customer is currently viewing, leveraging data from previous transactions and customer browsing behaviour to identify similar items.
For example, if a customer is looking at a makeup cleanser at Sephora’s website, the platform might recommend other makeup cleansers from different brands.
This helps customers explore a range of options, potentially leading them to discover product recommendations they might not have considered before.
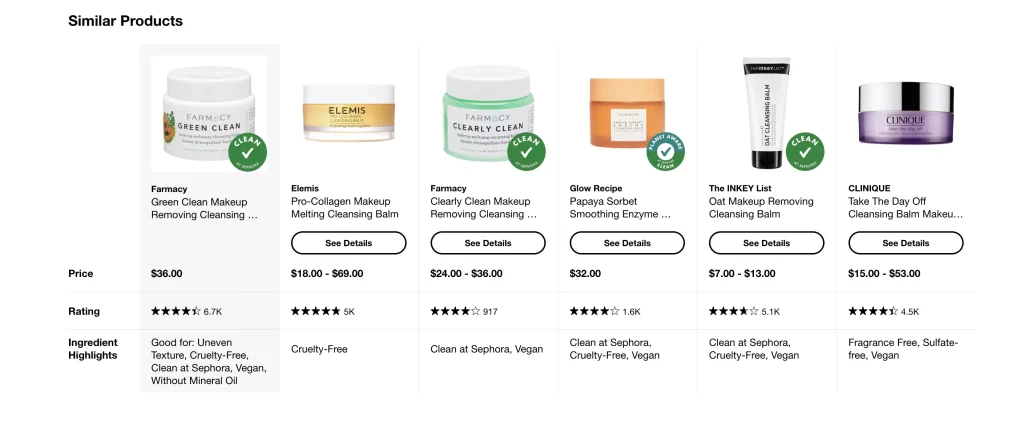
Pros and Cons
Pros: The feature offers several advantages, such as helping customers find product recommendations that fit their preferences more closely, thereby increasing customer satisfaction and potentially boosting sales by presenting more purchasing options
Cons: If not well-targeted, it can overwhelm users with too many options, leading to decision fatigue.
Newest Arrivals
Newest Arrivals product recommendations feature highlights the latest products added to their inventory to keep customers informed about the latest innovations and trends in their product lineup.
For example, if YETI releases a new line of insulated water bottles with advanced cooling technology, this feature will prominently display these items to customers.
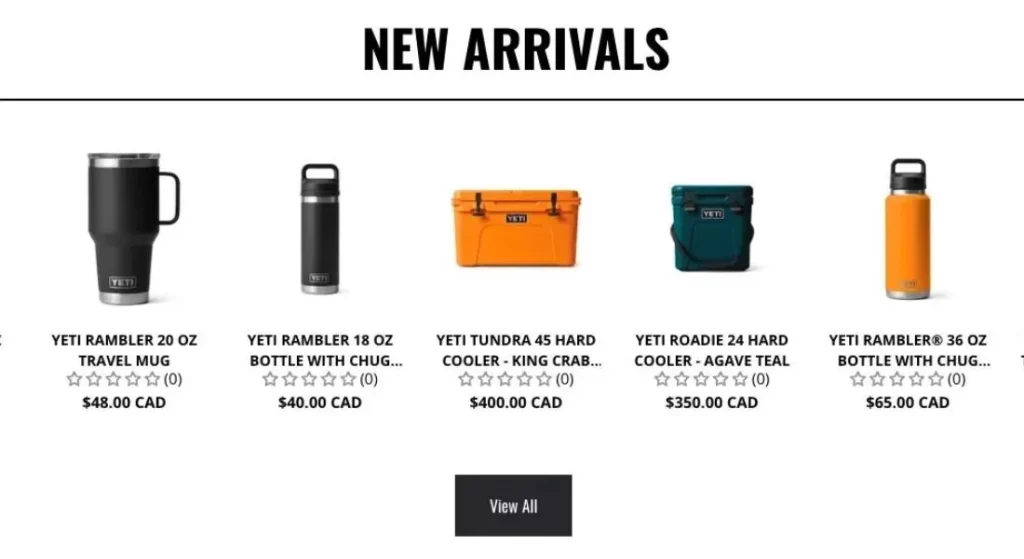
Pros and Cons:
Pros: Keeps customers informed about new offerings, stimulating interest and driving sales. Attracts trend-conscious customers.
Cons: May not be as effective for customers looking for tried-and-true products rather than new arrivals.
Trending Products
Trending Product recommendations feature showcases trending products on its platform to encourage users to opt for popular items.
For example, Netflix used this approach by creating a “trending” section to list movies or TV shows that are gaining rapid popularity, thereby attracting more viewers who are interested in what others are watching.
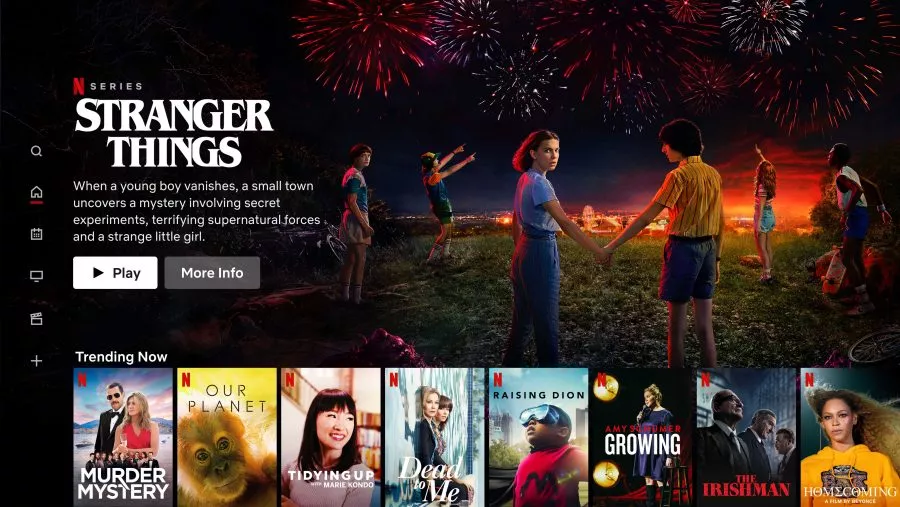
Pros and Cons
Pros: Leverages social proof to encourage customers to buy items that others are purchasing. Can boost conversions by creating a sense of urgency.
Cons: Trends can change quickly, requiring constant updates to maintain relevance.
Bestsellers
Bestsellers product recommendations feature highlights bestselling products on its homepage and product pages, showcasing items that have been popular with other customers.
For example, Barnes & Noble’s collects data of the novels that have consistent high sales, and features them in the “Bestsellers” section, making it more visible to potential buyers.
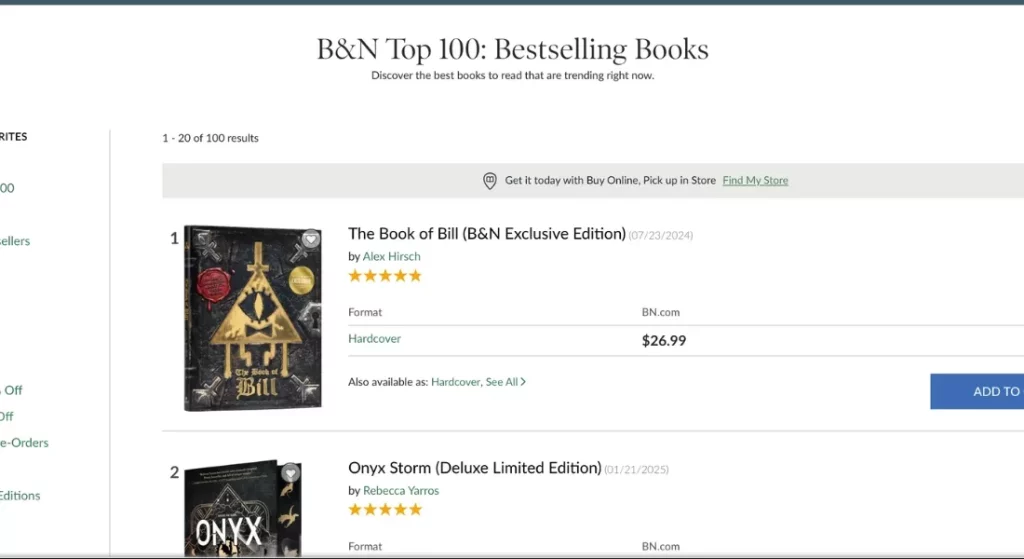
Pros and Cons
Pros: Showcases items validated by other customers, increasing trust and conversions. Appeals to customers looking for popular and well-reviewed products.
Cons: Over-reliance on bestsellers can overshadow other potentially good products that are not yet popular.
- Recently Viewed
Recently Viewed product recommendations feature reminds customers of items they recently viewed as they browse the site, helping them easily return to products they showed interest in.
For example, if a customer previously looked at a particular phone, Amazon will display it in the “Recently Viewed” section, making it easily accessible when they return to the site.
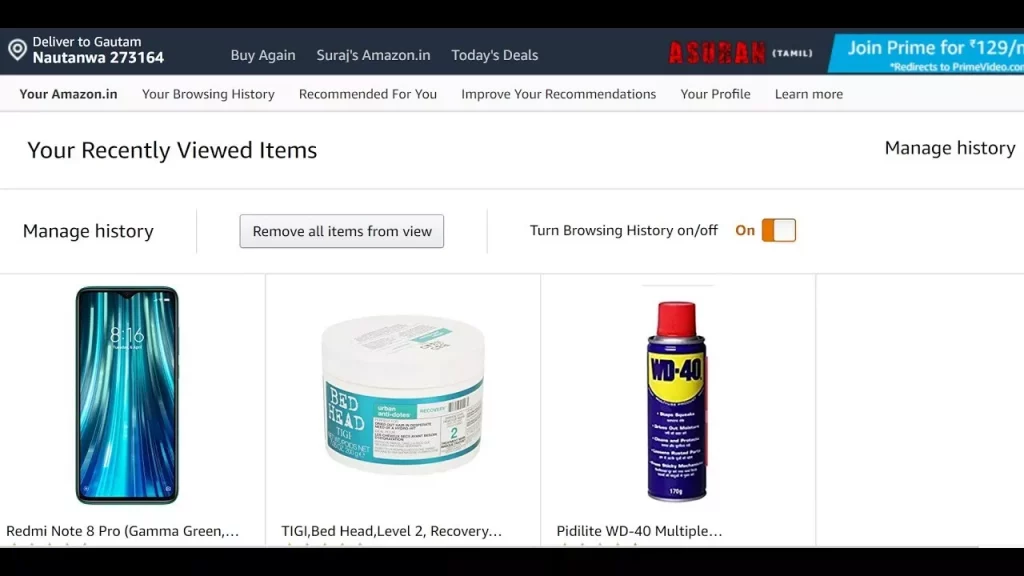
Pros and Cons:
Pros: Helps re-engage customers and guide them towards completing their purchase. Reduces cart abandonment rates by reminding users of their interest.
Cons: Can be less effective if customers are not interested in revisiting past viewed items.
Best Practices for Product Recommendations
Implementing effective product recommendation strategies is crucial for eCommerce success!
Here, we will guide you with some best practices to consider when designing and deploying your product recommendation engine:
1 . Use Data Effectively
Collecting and analysing data on customer behaviour, preferences, and purchase history is crucial for creating relevant product recommendations.
Leveraging machine learning algorithms can further enhance the accuracy and relevance of these suggestions by continuously learning and adapting to new data.
2. Personalise the Experience
Tailoring recommendations to individual users based on their unique behaviour and preferences significantly increases engagement and conversion rates.
Tools like Frizbit, which specialises in hyper-personalisation, can be particularly effective for implementing this strategy.
3. Provide Contextual Recommendations
Offering suggestions that are relevant to the current context, such as the product a user is viewing or their past interactions, can greatly enhance the shopping experience.
Using contextual clues like browsing history, location, and time of day helps refine these recommendations, making them more pertinent and timely.
4. Leverage Algorithms of Recommender systems
Collaborative filtering, which recommends products based on the behaviour of similar users, is a powerful tool for generating relevant suggestions.
Combining collaborative filtering with content-based filtering can create a more robust recommendation system, improving overall accuracy and user satisfaction.
5. Incorporate User Feedback
Allowing users to rate and review recommendations provides valuable feedback that can be used to refine and improve the recommendation engine.
This user-generated data helps ensure that the suggestions remain relevant and useful, enhancing the overall effectiveness of the system.
6. Optimise Placement and Timing
Strategically placing recommendations throughout the user journey—such as on product pages, homepages, and during checkout—maximises their impact.
Timing these recommendations to coincide with key decision points further enhances their effectiveness, driving higher engagement and conversions.
7. Test and Iterate
Using A/B testing to make data-driven decisions allows for continuous improvement of the recommendation system, ensuring it remains effective and relevant.
Ensure Transparency and Control
Being transparent about how recommendations are generated builds trust with users. Providing options for users to customise their recommendations and giving them control over their preferences, including the ability to opt-out, enhances user satisfaction and trust in the recommendation system.
Conclusion
By adopting these best practices and learning from successful examples, businesses can implement effective product recommendation systems that enhance customer experience, drive sales, and build brand loyalty.
If you want to learn more about his topic, make sure to read our Product Recommendation Engine for eCommerce – Ultimate Guide!
FAQ: Product Recommendations
Q: What is a product recommendation engine?
Product recommendation engines are systems that analyse different types of data — like product information, behavioural data, purchase histories, and more — to deliver relevant product recommendations to each individual customer
Q: What are the three main types of recommendation engines?
The three main types of recommendation engines are collaborative filtering, content-based filtering, and hybrid filtering.
Q:What is product recommendation in AI?
AI-powered product recommendations use algorithms and data to suggest the most relevant products to customers.
Q: What is an example of a product recommendation system?
- Amazon
- Netflix
- Spotify
Q: Which technique is used for product recommendation?
Collaborative filtering is a method used in recommendation systems to predict an individual’s preferences based on the preferences of similar users. The core concept is that users who have shared preferences in the past are likely to have similar tastes in the future.